Researchers at MIT and Takeda have found a way to make manufacturing pharmaceutical products much easier.
In pharma manufacturing, there is a need to isolate active pharmaceutical ingredient from a suspension and dry it, which requires a human operator to monitor an industrial dryer, agitate the material, and watch for the compound to take on the right qualities and compress them into medicine. Therefore, the job is reliant on the operator's observations.
What the Researchers Did
The researchers created a method to use physics and machine learning to categorize rough surfaces and characterize particles in a mixture. It uses a physics-enhanced autocorrelation-based estimator (PEACE) that could be a game-changer for manufacturing pills and powders, as reported by PhysOrg.
This could result in better efficiency and accuracy, lessening failed batches of Pharma products.
Their work is part of the ongoing collaboration between Takeda and MIT that launched in 2020.
Initially, the team planned to use videos to train a computer model to replace a human operator. However, determining which videos to train the model is too subjective.
Instead, the team decided to illuminate particles with a laser during filtration and drying. Then, they measure particle size distribution using physics and machine learning.
The researchers shine a laser beam on top of the drying surface and observe. With this, it doesn't need to stop and start the process, which means the job is more efficient and secure than the SOP.
Additionally, the machine learning algorithm also doesn't require many datasets to learn its job because the physics allows for fast neural network training.
This physics and machine learning method to measure particle size distribution significantly improved over the initial video plan. It eliminates the subjectivity of selecting videos and makes the process more efficient and secure.
Moreover, the fast training of the neural network due to physics reduces the need for a large dataset, making the process more cost-effective. Overall, this method is a promising development in particle size distribution measurement.
Read also: Pharmaceutical Leaders, Drugmakers Weigh in on Texas Abortion Pill Ruling
A Potential Game-Changer
Particle size distribution is essential in many industrial processes, including pharmaceuticals, chemicals, and food production. Determining the particle size distribution accurately can significantly affect product quality; hence, a reliable measurement method is crucial.
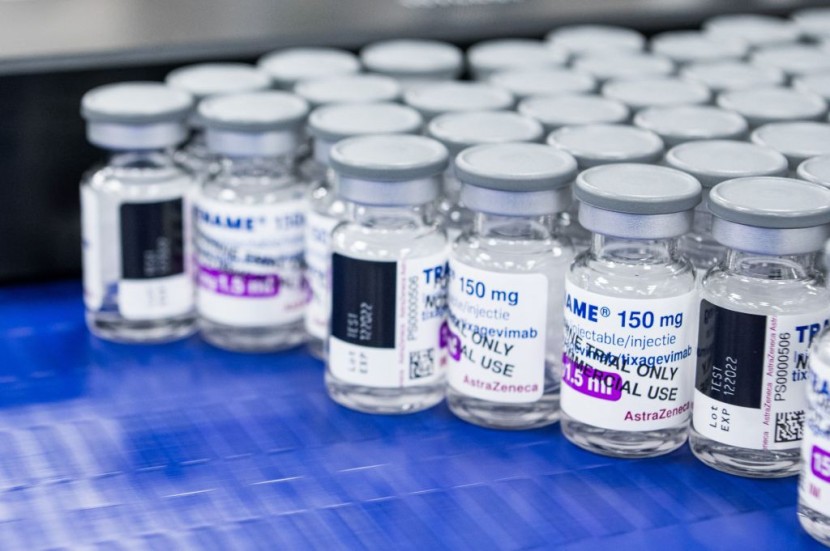
Using physics-based methods combined with machine learning algorithms provides one promising solution that eliminates some limitations associated with traditional approaches while improving accuracy levels.
Using lasers during filtration and drying stages instead of continually collecting samples through video recordings or other techniques involving manual intervention by operators like SOPs - this new approach reduces subjectivity from human influence on data collection procedures, making measurements more accurate than ever.
Moreover, Machine Learning algorithms powered by physics enable fast training, making them capable enough without requiring vast datasets compared with conventional neural networks used previously at larger scales- thus reducing costs incurred when researching these concepts further over long periods across various industries globally!
Related article: Turing Pharmaceuticals To Decrease Price Of AIDS-Related Drug Daraprim Following Backlash